The AI doppelgänger experiment – Part 2: Copying style, extracting value
💰 On training computers to see like capitalists
This is part 2 of a two-part series describing an experiment Sitong Wang (Columbia University) and I conducted over the past year. We invited four illustrators to use a generative model fine-tuned to their style (a task known as “style transfer”) and asked them what they thought of the results. We wanted to understand how artists see the output of such stylistic doppelgänger and how artists’ and machines’ ways of seeing style differ. If you haven’t already, go read Part 1 – The Training. You can also now read the paper we wrote about this study here.
Dear OnLookers,
Artists and machines, we know, have been competing for some years now. What winning looks like remains unclear, but the competition structure reveals much about who makes the rules. Rather than a wrestling match, the competition between artists and AI looks more like a race on parallel tracks. As their tracks unfold side by side, we make assumptions about the commonalities the contenders might share. After all, competition involves a certain degree of equivalency. The black box of AI is made equivalent to the black box of a person’s brain, models’ training is framed as comparable to human inspiration, and so on.
However, the ground on which these equivalences are drawn is rarely questioned. Instead, we take for granted that the disruptive impact of “AI” on the creative economy must mean that something necessarily creative is going on with it, or at least akin to creativity. With this unquestioned truth follows inaccurate comparisons, oversimplifications, and general fearmongering. Artists are left to either prove that what they do is somehow magical (as magical as AI is supposed to be), or worse, they have to compete in tech companies’ terms and try to be the cheapest, the fastest, etc.
What happens when we nudge these parallel lanes to intersect? When we turn the rat race into a boxing ring and allow artists to fight (In this case, look) back? We already have some examples of this in the direct confrontation by artists in class action lawsuits against AI companies or the design of countermeasures against web scrapping and style mimicry. In these disruptive moments, the rules of the game are challenged, and so is the inscrutability of machine learning.
The doppelgänger experiment we conducted contributes to these efforts. The experiment offered a space for artists to look closely and safely at a system usually built to see and consume their work while remaining invisible. Maybe surprisingly, illustrators found that whatever was generated by the model wasn’t their style and, at times, barely qualified as an illustration.
On the one hand, generative AI is disrupting the creative economy because it is assumed it provides something like artists' work yet on the other hand, artists find the results of these models to be far from successful. How, then, can we make sense of these two realities?
With this experiment, we suggest that the success of generative AI models in reproducing artists’ styles has little to do with a model’s performance and everything to do with who looks at it.
A quick overview of all the reasons artists were unimpressed by their AI doppelgänger
I won’t go into too much detail on the findings here, but if you’re curious, you can read the full paper here. Here is the bullet point version of our results:
Illustrators found the model successful at copying fragmented, isolated aspects of their styles. Bits of texture, shading, and color palettes were usually pretty good, but they were clear on one thing: they never amounted to “style.” That was interesting to find because when you read the computer science literature on style transfer, style is rarely, if ever, defined and is often used as a proxy for these isolated elements. This points to a substantial qualitative difference between artists’ and machines’ versions of style.
Style transfer is limited by what computer scientists call “content-style disentanglement,” which is the goal of training a model to separate the semantic content of an image from its style. However, what artists found is that their style is just as much about how they draw things as what they draw. Some never draw trees, others always draw birds, etc. Conversely, aesthetic elements in illustrations are meaningful semantically; changing the colors or the texture conveys different meanings. This is important because this shows how the problem with style transfer isn’t about the output's quality but about the systems' design.
While models are stuck with their datasets, a fixed snapshot of a corpus of images, artists’ styles evolve with each new image they create. Instead of a recipe to follow the same way every time, their style is an emergent quality of their artistic practice, which changes and adapts. When using the models, artists found little use to such a crystalized version of their style.
In sum, when artists were given a chance to examine a model’s output closely, they were unimpressed by its results. Not because of its quality but because of the model’s very logic about what style is, and what images are made of. If generative AI models only generate a fragmented, shallow copy of style, if it’s bound to fail because of the very design of separating content from aesthetics, and if style is an ever-emerging quality of creative practice that can’t be reduced to an algorithmic recipe to follow, then how come is generative AI poised to replace artists by generating images in any style?
The answer, unsurprisingly, has nothing to do with innovative technologies but everything to do with good ole’ capitalism.
Learning to see style like a capitalist
Style isn’t one thing, and neither is looking. The results I mentioned above were true when artists evaluated their doppelgänger but less so when they looked at the other participants’. In other words, artists were more likely to judge other people’s copies as successful than their own. There are many ways to interpret this, but this shows there’s no such thing as a style nor a universal way of seeing it; previous experiences, personal connection, etc, always guide our perception. For generative models, that vantage point, as we saw in our experiment, is not artists’. The question is, whose way of seeing style is performed by generative AI?
As I mentioned in Part 1, paying attention to illustrators’ perspectives makes it impossible to talk about art without talking about the economy. While AI companies are eager to hide the economics of their system to focus on the PR discussion of “democratizing creativity,” illustrators are quick to oppose this blind spot.
As I sat with these illustrators to conduct the experiment, our conversations digressed beyond what was happening on the screen. Confronting generative AI’s ways of seeing was a gateway to think about all the other ways of seeing one’s work that make up the creative industries. We discussed plagiarism, moodboards, intellectual property laws, contracts, social media, etc. This is the context in which style transfer and generative AI unfold. Legal strategies like work-for-hire contracts or NDAs strip away the ability for artists to retain control over their work, design practices like moodboards extract styles from their sources without keeping track of who makes what, and in-house design teams can copy any illustrator’s work without hiring them as style isn’t protected by copyright.
The industry was already structured to objectify, extract, and capitalize on styles long before generative AI arrived. Artists have long dealt with such ways of seeing from clients eager to extract value from their work. Style transfer, and generative AI in general, doesn’t democratize creativity; it optimizes ways to capitalize on it. And seeing style from a style transfer perspective means seeing it from a capitalist perspective.
Copying styles, extracting value
At the end of an interview, one participant reflected on how clients’ affinity with AI is clear even when they work with human artists: “I do get these briefs of people like this, the client who wants me to do like 30 images in two weeks plus animation, I think they would love to just generate something […].” The shallow understanding of style and artworks that we saw AI perform, while so different from what artists do, matches clients’ desire for cheaper, faster, more docile ways to make money from their work. As this illustrator concludes, “You want like a nice image and a specific style, then kind of prompting me like an AI”. As I’ve argued elsewhere, AI and the creative industry were a match made in capitalist heaven.
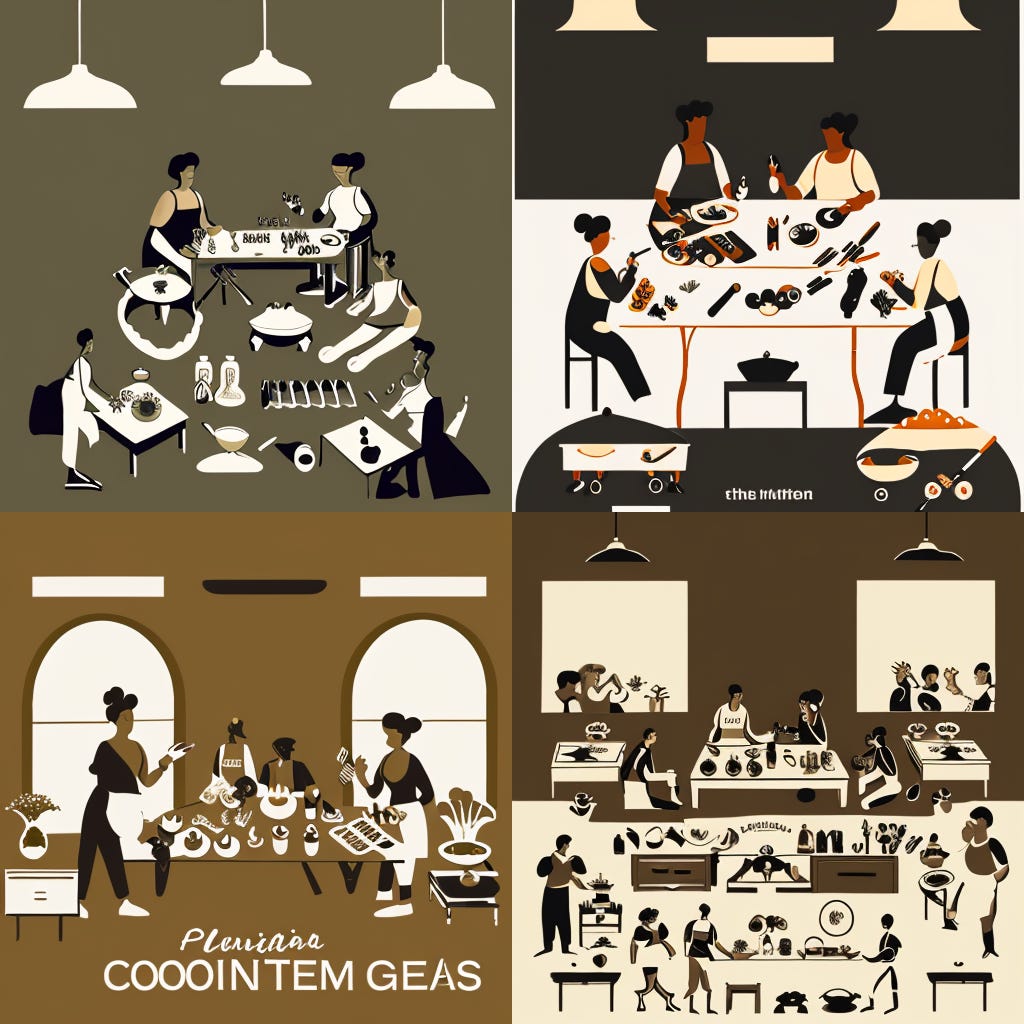
The “styles” generated by AI models closely follow the market logic of extracting value from artwork without dealing with the artist. They are fragmented, meaning clients can choose which parts to keep or discard, regardless of the whole. They rely on separating content from aesthetics, allowing marketing executives to brainstorm (usually bad) ideas and only use style as a superficial, disconnected layer to slap onto them. They are crystalized snapshots in a repertoire rather than emerging, evolving features of creative practice, which makes them wieldier and more docile than unpredictable artists’ styles.
Computer scientists often thought they were aligned with artists’ ways of seeing, while in practice, they trained their systems to see like a client. Frequently taunted as a “creativity support tool” that democratizes creativity (cf.
’s good piece on this), this experiment suggests that generative AI models can also (and more accurately so) be understood as a supply-chain optimization one. If there’s a form of creativity generative AI supports, it is that of managers endless imaginings of new ways of extracting value from people’s labor.Technology doesn’t happen in a vacuum, and it’s impossible to understand the performance of a model without talking about the specific context in which it unfolds. Training a model to interpret images is training it to see from a vantage point. Our experiment has shown that rather than a universal perspective on style and images, let alone the perspective of artists, it is an extractivist vision of style aligned with corporate interest that is embedded in generative AI.
The same output can be considered terrible by an artist and yet great by a client. But that doesn’t mean what artists and AI do are similar; it means that clients lack the visual literacy to tell the difference. Whether it’s telling designers how to design based on website traffic data or replacing illustrators with AI-generated images, Big Data-backed management seems to think it can cut on costs by ignoring the expertise and taste of creatives.
By reintegrating art within its political and economic context, we gain a new lens through which to evaluate the performance of generative AI. Suddenly, style transfer is no longer about copying artists' essence but about extracting value from their labor. As we wrote in the paper: “Generative AI in general, and style transfer in particular, have often been praised as innovative technological solutions, but for illustrators who try to make a living from their art, they mostly carry on old capitalist problems.” As machines learn to see images in a certain way, artists (and everyone really) must, in turn, learn to identify, question, and oppose these ways of seeing.
P.S. Hello to all the new readers around here (thanks to a recent interview I did with the New York Review of Books), I hope you’ll browse through the archive as well as find future essays worth reading!
"Computer scientists often thought they were aligned with artists’ ways of seeing, while in practice, they trained their systems to see like a client." Yes yes yes!
Brilliant! Walter Benjamin lives on in the way you think.
Another step you might take in your research: have artists and also non-artists examine a mixture of AI-generated images and original images. Can they tell which is which?